Climate Change: Quantifying the Poverty Impact of the 2022 Floods in Pakistan
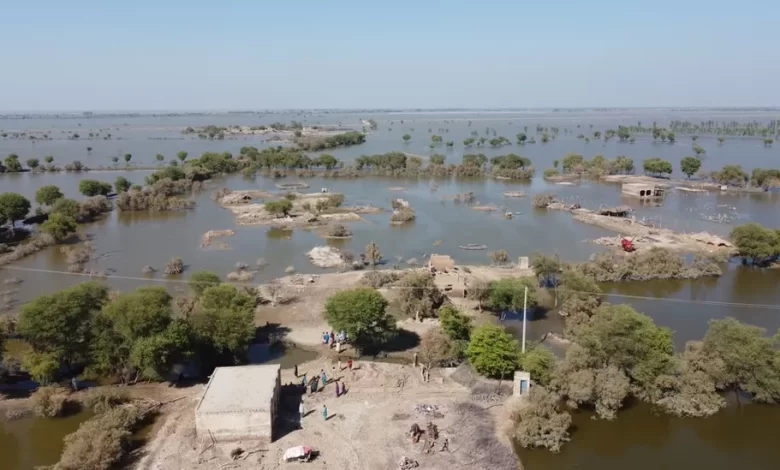
Exacerbated by climate change, the 2022 Monsoon Floods in Pakistan severely affected the lives and livelihoods of millions of people, especially in the Sindh and Balochistan provinces. The floods particularly impacted the poorest and most vulnerable, who are disproportionately more likely to live in flood-affected areas. Pakistan’s Post Disaster Needs Assessment estimates suggest that the floods may have led as many as 9.1 million people–or four percentage points–into poverty. This work was featured by the government of Pakistan as part of its advocacy for ‘loss and damages’ at COP 27.
How then were those estimates constructed?
Measuring poverty in real-time
Quantifying the poverty impact informs the humanitarian response and the allocation of resources during the recovery. It also highlights the relevance of sub-national patterns because natural disasters are often geographically concentrated and disproportionally affect specific segments of the population—notably farmers in rural areas.
In an emergency, the limited availability of real-time data on the ground poses a particular constraint requiring innovative approaches. The availability of satellite data overlaid with household survey data allows for rapid estimates of the extent and severity of the flooding. This combination of data allowed us to calculate how the damage impacted people and, from there, derive an estimate of the increase in poverty. These estimates were later validated using data collected by the government in flood-affected districts.
Leveraging geospatial data
Within days, multiple space agencies published satellite data of the fluvial flooding. These high-resolution images showed the geographic extent of the flooding, highlighting large parts of the country along the southern Indus River, which were underwater (figure 1).
Figure 1: Flood Extent
This remotely sensed maximum flood extent closely resembles the probabilistic scenarios representing events with a frequency of 1 in 100 and 1 in 1,000 years floods, presented in the recent Pakistan Climate Change and Development Report (CCDR). We combined this flood layer together with other geospatial information, such as population distribution and indicators of economic activity, to calculate their exposure to floods. The spatial modeling of the population comes from the 2019 World Settlement Footprint.
Integrating household data
The impact of floods on household welfare is measured by various factors. These factors include the location of the household, the percentage of homes, land, and livestock exposed to floods within that district, and household characteristics that help determine the likelihood of being impacted by floodwater. The satellite data on flood exposure were combined with data from the 2018-2019 Household Income and Expenditure Survey (HIES), the source used to calculate official poverty figures. The resulting combined data confirmed that the calamity-affected districts were poorer than the national average, with an average poverty rate of 31.4 percent compared to 21.9 percent nationally.
Merging the dataset allowed the estimations to consider household characteristics when assessing the damages. Households who worked in agriculture would be exposed to losses to their livelihoods since arable land was underwater. Households who lived in mudbrick homes would be more likely to see them washed away by the floods. Households with livestock would risk losing their animals and, therefore, an essential source of income. These risks were factored into a model that ‘shocked’ affected households, reducing their consumption based on a damage function. We iterated 500 times to reduce the potential bias from assigning damages to households based on probabilities.
Finally, to factor in the effect of disruptions to the supply chain, the model factored in how price increases could have affected food consumption. This was done using the monthly Consumer Price Index reported by the Pakistan Bureau of Statistics. Losses to household welfare were proportional to the total consumption dedicated to food.
Validating with Government reports
This model was validated against reports from the National Disaster Management Agency (NDMA). Official estimates of the population, buildings, land, and livestock exposed were substituted for the geospatial estimates to rerun the model.
The results were closely aligned (figure 2). Effects on poverty are spatially concentrated in calamity-hit provinces; affected households had overlapping characteristics, making them vulnerable to losses through various channels. The exception is Balochistan, where the government reports a larger share of the population being affected by flooding compared to what is suggested by the geospatial data. An explanation for this could be that satellite images could capture standing water but could not detect quick pluvial cumulation and flash floods that likely caused large damages in this region.
Figure 2: Estimated increase in the national poverty rate – comparing geospatial analytics and NDMA estimates
NDMA results were used to construct the official estimates of the impact on poverty, which were between 3.7 and 4 percentage points, or about 9 million people. The depth of poverty (distance to the poverty line) increased as well, with an estimated 7 million additional people living more than 20 percent below the poverty line. These are short-term estimates, and the recovery will depend on the quality of relief and reconstruction efforts. Even in the best case, reversing these adverse shocks to household welfare will take considerable time.
Data-intense assessments will be needed even more in the future
Climate change projections suggest that natural disasters such as floods may become increasingly severe and frequent in the following decades. Projections from the CCDR suggest that due to these cumulative shocks, hard-won gains in terms of poverty reduction may stall or be reversed.
Through this work, it has become clear that it is essential to continue strengthening our capacity to align poverty estimates based on geospatial, administrative and survey data. It also highlights the importance of regularly collected household survey data both before and after the shock. This can help policymakers identify potential risks that households and communities face as the climate evolves. It can also provide insights on how to help them adapt and be more resilient. And when a shock occurs, such analysis can help assess the damage quickly and deploy support more effectively.